Computational Psychiatry
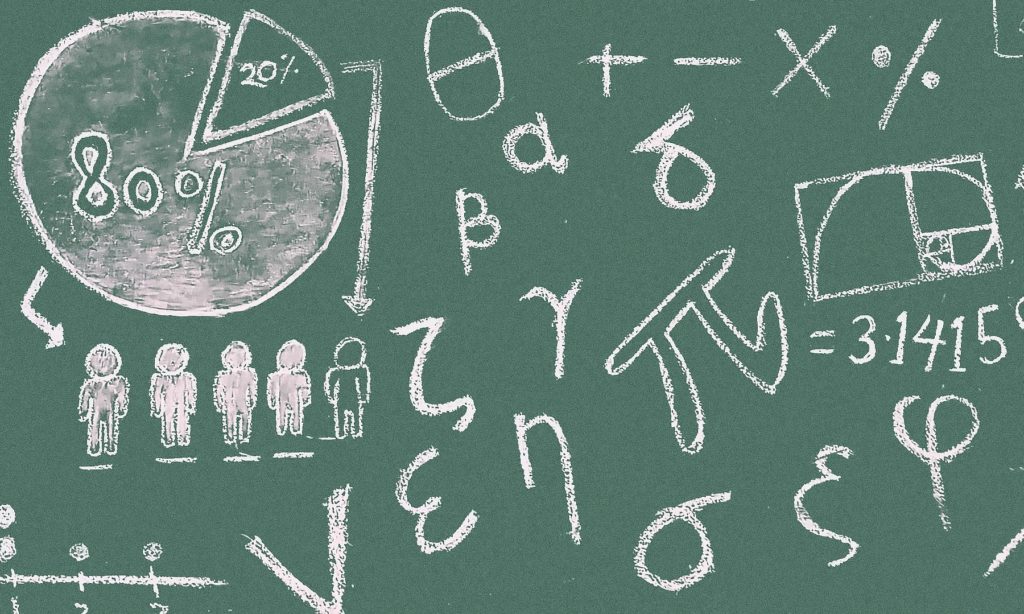
This post summarizes “Computational Psychiatry” written by Montague, Dolan, Friston, and Dayan published in Trends in Cognitive Science in January 2012. The article PDF is attached.
Computational Psychiatry
The goal of computational psychiatry is to quantitatively define problems in psychiatric disease and to characterize mental dysfunction in terms of aberrant computations. Future research needs to focus on theory development and large-scale computational phenotyping in human subjects.
The Explanatory Gap
An explanatory gap exists between psychiatry and neuroscience, that is, between the phenomenological and the molecular. This gap is reflected in the concerns regarding the classifications of psychiatric diseases in the DSM. Advances in neuroscience can begin to bridge the gap. Aberrant decision-making offers an opportunity for progress in treating the majority of psychiatric illness.
Mathematical Modeling
Mathematical modeling is a quantitative expression of natural phenomena using multilevel reductive descriptions. This biophysical realism is difficult to achieve with incomplete data. For example it is not practical to build a biophysical model of a single neuron as the synaptic responses can only be measured using an average of billions of other neurons using fMRI or EEG.
Biophysical modeling is important for understanding the relationships within a huge complex system. For example, dopamine, serotonin, norepinephrine, and acetylcholine are all subject to intricate patterns of feedback and control. Biophysical modeling can predict these complex interactions. These predictions are not equivalent, however, to computational modeling.
Computational Modeling
The goal of computational modeling is to define normative computational accounts of neural and cognitive function. The brain is organized to maximize or minimize quantities of external and internal importance.
Computational Modeling in Decision-making
A subfield of computational modeling is decision-making. Decision-making is choosing a possible option based on the accumulation of evidence. Many psychiatric conditions are associated with aberrant decisions. For example, individuals with depression make the choice not to explore; individuals with obsessive compulsive disorder choose to endlessly repeat a behavior; individuals with drug addictions continue to use harmful substances. In sum, “if the psychology and neurobiology of normative decision-making can be characterized and parameterized via a multi-level computation framework, it will be possible to understand the many ways in which decision-making can go wrong.”
Early Connectionist Models of Mental Dysfunction
Connectionism is the perspective that the brain is a network of neurons connected by synapses and that complex functions emerge from networked interactions of simple parts.
Recent Efforts Toward Computational Characterization of Mental Dysfunction
The authors believe that future research must focus on extracting normative computations accounts of both healthy and pathological cognition with the goal to build predictive models of individuals. The authors assert that achieving this goal will require large scale computational phenotyping of human behaviors which currently does not exist.
Reinforcement Learning Models of Mood Disorders and Anxiety
There are three different types of control systems in reinforcement learning: (1) model-based, (2) model-free, and (3) Pavlovian. Model-based and model-fee links actions to affective consequences. Pavlovian control system, on the other hand, determines involuntary actions based on predictions of outcomes.
Computational modeling uses these control systems to explain depression. Animal studies suggest that serotonin release is involved in learned behavioral inhibition and prediction of aversive outcomes. This reflects a Pavlovian effect: patients with depression (who theoretically lack serotonin release) never learn what to do if faced with a future hardship. This effect is termed the “serotonin crutch.” Additionally, model-based reinforcement learning captures learned helplessness.
Using Games to Phenotype ASD and BPD
Theory of mind is a defining aspect of human cognition. Individuals with autism spectrum disorder possess a diminished capacity for socio-emotional reciprocity. Game theory is defined as, “the study of mathematical models of interacting rational agents.” Two game approaches have been used to study ASD and other psychiatric illnesses: (1) stag hunt game and (2) multi-round trust game. Both games encourage the human player to make inferences about their partner’s mental state during the game. The stag hung came has been used to estimate theory of mind in patient with ASD. The multi-round trust game has been used to study BPD and measure “fairness sensitivity.”
Download the Computational Psychiatry PDF
Share on Facebook Share on Twitter Share on Pinterest
0 Comments on "Computational Psychiatry"